Introduction
Hi, my name is Nghi Le. I’m a Data Science major with a minor in Music. I have a deep passion for both Math and Music, and my capstone project is a unique blend of these fields.
Abstract
Existing emotion-based Music Recommendation Systems (MRSs) have remained underdeveloped due to the lack of focus on users’ emotion approaches and the inherent psychological complexity of human emotions. To address this gap, this research introduces a novel recommendation framework that integrates users’ emotional states into the music recommendation process. Specifically, the system first employs a facial expression analysis (DeepFace) for real-time detection of user emotions, then translates these emotions to scientifically validated musical attributes using Music Emotion Recognition (MER) tags guided by the Circumplex Model of Emotion. Finally, content-based filtering techniques are utilized to generate personalized playlists tailored to the user’s current emotional state. Experimental results demonstrate that the proposed framework successfully delivers emotion-responsive music recommendations, effectively catering to both users’ emotional needs and musical preferences.
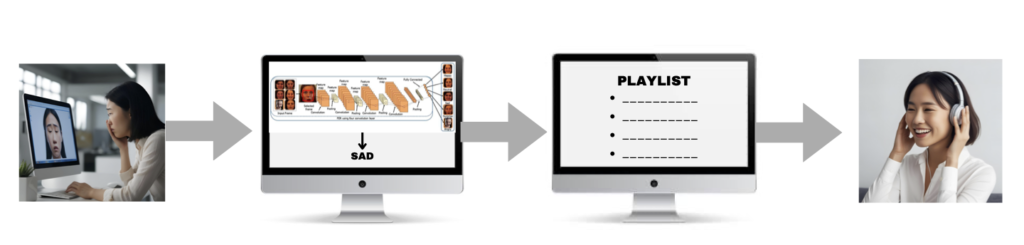
Data Architecture Diagram
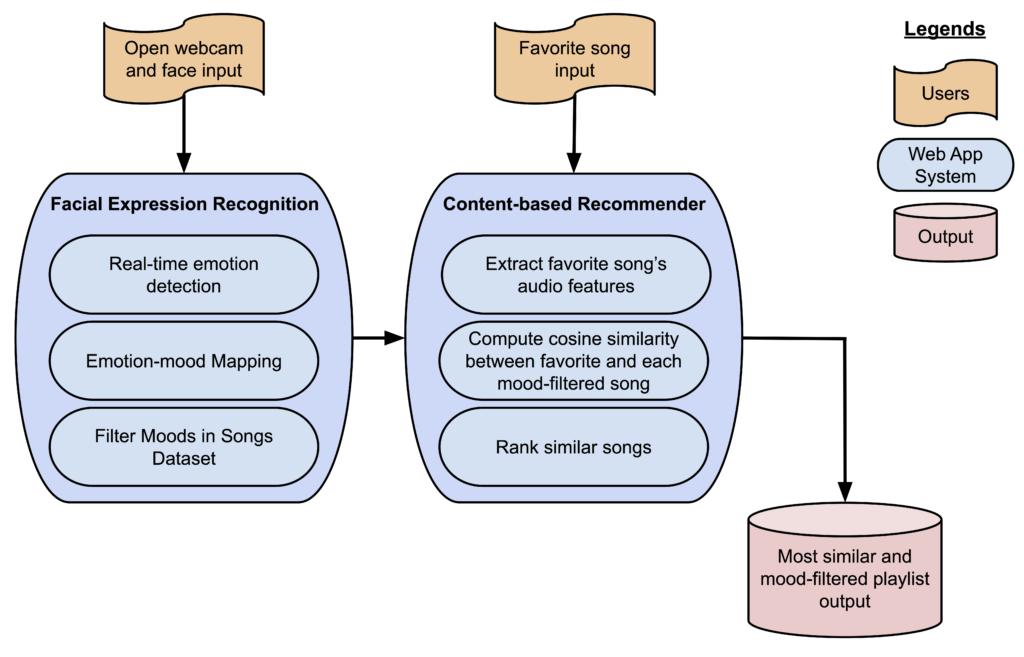
Poster
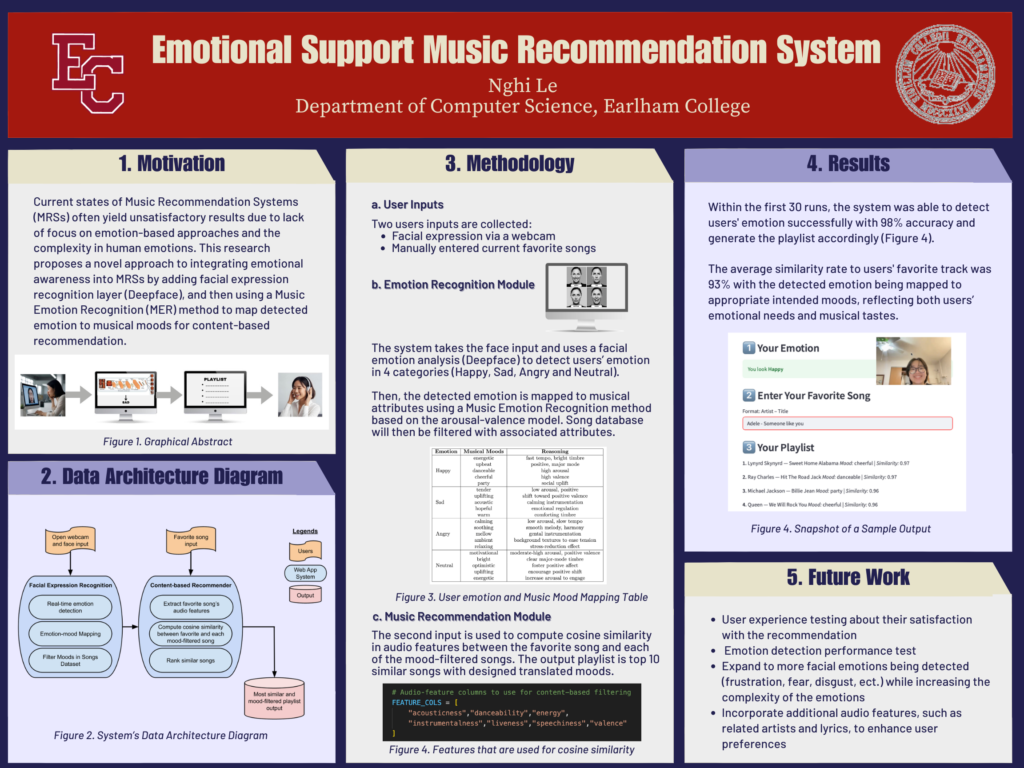